Elon Musk, renowned entrepreneur and tech visionary, has joined the growing consensus among artificial intelligence experts that the era of relying solely on real-world data for training Artificial Intelligence models is coming to an end. Speaking during a live-streamed conversation with Stag well chairman Mark Penn on X, Musk made a bold statement: the pool of human knowledge available for Artificial Intelligence training has been effectively exhausted.
“We’ve now reached the point where we’ve essentially tapped into the cumulative sum of human knowledge for Artificial Intelligence training,” Musk revealed during the livestream. “This significant milestone was essentially crossed last year.” His insights align with the remarks of Ilya Sutskever, the former chief scientist at Open AI, who previously addressed this challenge at the prestigious NeurIPS machine learning conference. Sutsk ever introduced the term “peak data,” emphasizing the impending shortage of real-world data and predicting a paradigm shift in Artificial Intelligence development methodologies.
Table of Contents
ToggleSynthetic Data The Emerging Solution to Peak Data Challenges
Faced with the dwindling availability of real-world training data, Musk has proposed synthetic data as the logical next step for Artificial Intelligence development. Synthetic data, created by Artificial Intelligence models themselves, offers a path forward by generating datasets that mimic or extend real-world scenarios. According to Musk, “The only viable way to supplement existing data is through synthetic data, where Artificial Intelligence systems generate their own training datasets. This self-learning approach enables models to evaluate, refine, and iterate on their outputs in a closed-loop system.”
Musk’s endorsement of synthetic data reflects a broader trend in the Artificial Intelligence industry. Leading technology giants such as Microsoft, Meta, Google, Open Artificial Intelligence, and Anthropic are already leveraging synthetic datasets to enhance their advanced Artificial Intelligence systems. Synthetic data has played a crucial role in the development of notable AI models like Microsoft’s Phi-4, Google’s Gemma models, Meta’s Llama series, and Anthropic’ s Claude 3.5 Sonnet. These companies use synthetic data alongside real-world datasets to train and fine-tune their cutting-edge systems, highlighting its growing importance in Artificial Intelligence development.
Advantages of Data in Artificial Intelligence Development
The adoption of synthetic data offers several notable advantages, making it a pivotal resource for the Artificial Intelligence industry. One of the most significant benefits is cost-effectiveness. Artificial Intelligence startup Writer, for example, successfully developed its Palmyra X 004 model using almost entirely synthetic data for a fraction of the cost required for traditional methods. The company’s investment of $700,000 stands in stark contrast to the $4.6 million typically required for comparable models developed by industry leaders like Open Artificial Intelligence.
In addition to cost savings, synthetic data enables researchers to simulate rare, complex, or even hypothetical scenarios that may be challenging—or impossible—to capture in real-world settings. This capability opens new frontiers for Artificial Intelligence applications, allowing for advancements across diverse industries, including autonomous vehicles, healthcare, financial modeling, and robotics.
Moreover, synthetic data offers scalability, allowing Artificial Intelligence developers to generate vast quantities of data tailored to specific tasks or environments. This scalability not only accelerates the Artificial Intelligence development process but also ensures that models are trained on datasets relevant to their intended applications.
Challenges and Risks of Synthetic Data Adoption
While synthetic data holds great promise, it is not without its challenges. One of the most pressing concerns is the risk of “model collapse,” a phenomenon where Artificial Intelligence systems trained predominantly on synthetic datasets lose their ability to produce creative, unbiased, and high-quality outputs. Over time, this reliance can lead to diminished functionality and the amplification of biases present in the synthetic data itself.
Musk and other experts have highlighted this potential pitfall, noting that synthetic data inherits the biases, errors, and limitations of the Artificial Intelligence models that generate it. If these issues are not addressed, the outputs of Artificial Intelligence systems trained on synthetic data could perpetuate inaccuracies and inequities, undermining their reliability and real-world applicability.
A Transformative Shift in the AI Landscape
The industry’s pivot to synthetic data marks a transformative moment in the evolution of artificial intelligence. Gartner estimates that by 2024, approximately 60% of the data used in AI and analytics projects was synthetically generated. This shift underscores the increasing reliance on artificial data as a cornerstone of Artificial Intelligence development and reflects a growing recognition of its strategic value.
For leading technology companies, synthetic data represents a means to overcome the limitations of real-world datasets while maintaining their competitive edge. By harnessing the power of Artificial Intelligence-generated data, these organizations are poised to drive innovation, enhance efficiency, and expand the capabilities of their models across diverse applications.
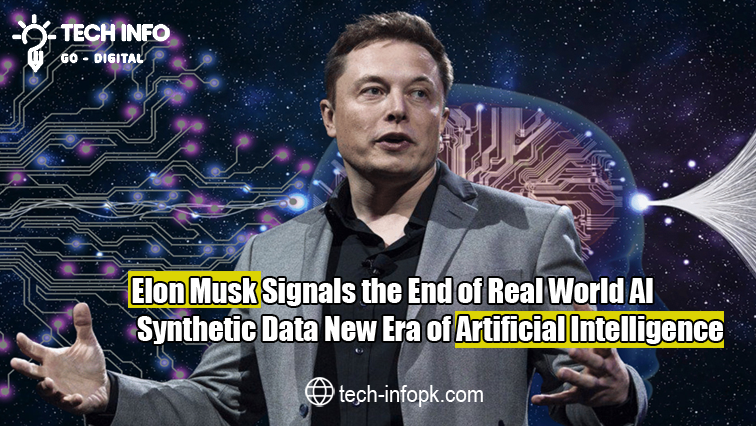
Conclusion
Elon Musk’s acknowledgment of the exhaustion of real-world training data highlights a pivotal juncture in the artificial intelligence industry. As the availability of traditional datasets diminishes, synthetic data emerges as a vital resource, offering a path forward for Artificial Intelligence development.
While synthetic data presents unparalleled opportunities for cost reduction, scalability, and innovation, it also requires careful management to mitigate potential risks such as model collapse and bias amplification. The industry must adopt robust safeguards, ethical guidelines, and rigorous testing protocols to ensure the reliability, fairness, and accuracy of Artificial Intelligence systems trained on synthetic data.
As companies like Microsoft, Google, Meta, and Open Artificial Intelligence continue to refine their approaches, synthetic data is set to play an increasingly central role in shaping the future of Artificial Intelligence. By striking a balance between innovation and responsibility, the industry can unlock new possibilities, addressing complex challenges and creating transformative solutions that drive progress in business, science, and society.