In the competitive world of artificial intelligence, the release of groundbreaking models is often accompanied by scrutiny and intrigue. Recently, DeepSeek, a well-funded Chinese AI laboratory, unveiled its latest creation, DeepSeek V3, an “open” AI model that has garnered attention for outperforming many rivals in popular benchmarks. This large yet efficient model is designed to excel in text-based tasks, including coding, content generation, and essay writing. However, a peculiar issue has emerged — DeepSeek V3 seems to mistakenly identify itself as OpenAI’s ChatGPT.
Table of Contents
ToggleThe Phenomenon of Misidentification DeepSeek V3’s ChatGPT
A series of tests and observations, including posts on social platforms like X (formerly Twitter), reveal that DeepSeek V3 often claims to be ChatGPT, specifically identifying itself as OpenAI’s GPT-4 model released in 2023. In 5 out of 8 test scenarios, the model insisted it was ChatGPT (v4), while correctly identifying itself as DeepSeek V3 in only three instances.
This misidentification extends beyond mere self-referencing. When asked for details about DeepSeek’s API, the model reportedly provides instructions for OpenAI’s API instead. Furthermore, DeepSeek V3 reproduces some of the same jokes and punchlines as GPT-4, sparking discussions about the potential overlap in their training data.

Exploring the Root Cause
Understanding this phenomenon requires a closer look at the architecture and training methodologies of generative AI models like ChatGPT and DeepSeek V3. These systems are essentially statistical engines trained on massive datasets. By analyzing billions of examples, they learn patterns and correlations to predict the next sequence of text based on a given prompt.
The peculiar behavior of DeepSeek V3 could stem from its training data. While DeepSeek has not disclosed specific details about the datasets used, the presence of text generated by ChatGPT or GPT-4 in public datasets could explain this overlap. If such data were included in DeepSeek V3’s training set, the model might have inadvertently memorized GPT-4’s outputs and begun replicating them, including the self-identification patterns.
Challenges of AI Training Data Contamination
The issue of AI models misidentifying themselves or providing incorrect references highlights a broader challenge in the AI industry: training data contamination. As the internet increasingly becomes saturated with AI-generated content, filtering and curating high-quality training data has become more difficult.
By some estimates, 90% of the web could consist of AI-generated content by 2026. This flood of AI-created material, often referred to as “AI slop,” complicates the training of new models. Content farms, bots on social media platforms, and automated tools generating clickbait contribute to this growing problem. As a result, even rigorous dataset curation efforts may inadvertently include AI outputs, leading to situations like the one observed with DeepSeek V3.
Ethical and Technical Implications
Training AI models on outputs from rival systems raises ethical and technical concerns. Mike Cook, a research fellow at King’s College London specializing in AI, likens this practice to “taking a photocopy of a photocopy.” He explains that each iteration loses more detail and connection to reality, potentially degrading the quality of the model’s predictions and exacerbating inaccuracies.
Furthermore, this approach might breach the terms of service of the original AI systems. OpenAI, for example, explicitly prohibits the use of its products’ outputs to develop competing models. While it remains unclear whether DeepSeek intentionally used ChatGPT outputs in training, the possibility underscores the need for greater transparency in dataset sourcing.
Responses from Industry Stakeholders
Neither OpenAI nor DeepSeek has issued official statements regarding the situation. However, OpenAI’s CEO, Sam Altman, appeared to comment indirectly on the matter, emphasizing the challenges of true innovation in AI development. when you don’t know if it will work.”
This sentiment underscores a broader industry concern about the balance between leveraging existing technologies and fostering original breakthroughs.
Broader Implications for AI Development
DeepSeek V3 is not alone in misidentifying itself. Other prominent models, such as Google’s Gemini, have exhibited similar behavior, sometimes claiming to be competing systems. For instance, Gemini has reportedly identified itself as Baidu’s Wenxinyiyan chatbot when prompted in Mandarin.
These incidents point to a deeper systemic issue: the pervasive influence of AI-generated content on model training. Without effective measures to filter and validate training data, AI systems risk becoming less reliable and more prone to errors.
Cost-Saving vs Quality in AI Development
Heidy Khlaaf, Chief AI Scientist at the nonprofit AI Now Institute, highlights the appeal of cost-saving strategies in AI development. By “distilling” knowledge from existing models, developers can significantly reduce training costs. However, this approach is not without risks. Khlaaf explains that distillation from models like GPT-4 might lead to outputs that mirror OpenAI’s style and content, raising questions about originality and quality.
Even accidental exposure to GPT-4 data during training could result in a model like DeepSeek V3 exhibiting behavior reminiscent of ChatGPT. While such overlap might enhance certain capabilities, it also increases the likelihood of perpetuating existing biases and inaccuracies in the original data.
Addressing the Challenges
The industry must adopt robust strategies to mitigate these challenges. Transparency in data sourcing, adherence to ethical guidelines, and investment in advanced data filtering techniques are essential. Collaboration between AI companies, policymakers, and researchers can help establish standards that promote innovation while safeguarding quality and integrity.
The Allure of Imitation
The appeal of copying lies in its relative simplicity. Once a model like OpenAI’s GPT-4 proves its effectiveness, competitors are naturally drawn to emulate its architecture, training methodologies, or functionality. By mimicking proven strategies, organizations can save time, resources, and effort that would otherwise be required to build a model from scratch. This approach often results in faster deployment and lower risk of failure, making it an attractive proposition for firms looking to capitalize on the booming AI market.
However, this method is not without significant drawbacks. While replication might yield short-term benefits, it fails to push the boundaries of what AI technology can achieve. It creates a cycle of redundancy, where AI models compete not on originality or utility but on marginally enhanced versions of the same fundamental design. This not only limits the scope of innovation but also creates a saturated market filled with indistinguishable solutions.
The Risks of Playing it Safe
Altman’s statement emphasizes the risks associated with playing it safe in AI development. True innovation requires a willingness to embrace uncertainty and explore uncharted territories. Pioneering new ideas is inherently risky, as there are no guarantees of success. The road to groundbreaking innovation is often fraught with challenges, from technical obstacles to resource constraints and market skepticism.
Yet, it is precisely this willingness to take risks that drives technological advancement. Without experimentation and bold thinking, breakthroughs in AI would stagnate. The current success of models like GPT-4 and DeepMind’s AlphaFold is a testament to the value of risk-taking. These models did not emerge from copying existing technologies but rather from years of rigorous research, trial and error, and a commitment to pushing the boundaries of what AI could achieve.
Ethical Implications of Copying
Altman’s remarks also touch on the ethical dimensions of imitation in AI. When organizations replicate successful models without permission or attribution, they risk violating intellectual property rights and ethical guidelines. For instance, training an AI model on outputs generated by a rival system, such as GPT-4, raises questions about the integrity of the development process.
Such practices not only undermine trust in the AI industry but also pose broader risks to the ecosystem. Models built on unoriginal foundations may perpetuate biases, inaccuracies, or limitations present in the original data. This can lead to a degradation of quality across the industry, as successive iterations of copied models become increasingly detached from the foundational research that made the originals successful.
The Challenge of Originality
Developing something truly original, as Altman suggests, is far more demanding. It requires a deep understanding of AI principles, substantial investment in research and development, and a willingness to experiment with new approaches. Originality often entails working on concepts that have no precedent, making it difficult to predict outcomes or measure success in conventional terms.
Despite these challenges, originality is the cornerstone of progress. It paves the way for innovations that redefine industries and solve problems previously thought insurmountable. For example, OpenAI’s decision to release GPT-3 and GPT-4 as advanced language models has revolutionized natural language processing, enabling applications ranging from creative writing to complex problem-solving. These breakthroughs would not have been possible without the bold decision to invest in untested ideas.
A Call to Action
Altman’s statement serves as both a critique and a call to action for the AI community. It challenges developers, researchers, and organizations to prioritize originality over convenience. While copying might offer a shortcut to short-term gains, it is only through risk-taking and innovative thinking that the industry can achieve meaningful progress.
For aspiring AI developers, Altman’s words are a reminder of the importance of curiosity, creativity, and perseverance. The field of AI is still in its infancy, and there is immense potential for new ideas to shape its trajectory. By focusing on originality and embracing the challenges that come with it, developers can contribute to a future where AI is not just a tool for automation but a transformative force for good.
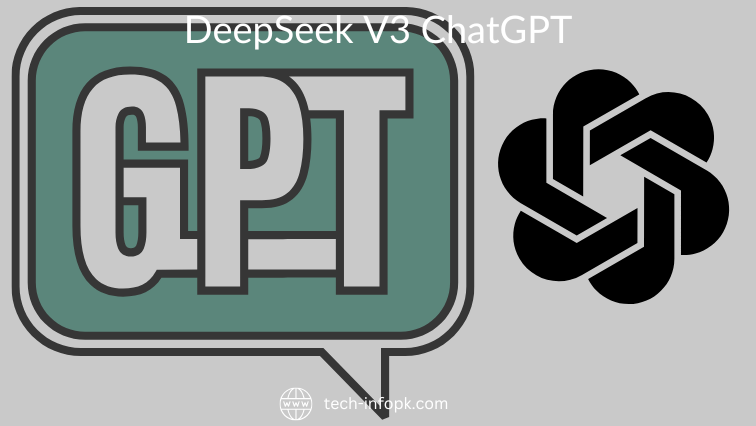
Conclusion
The case of DeepSeek V3 underscores the complexities of AI development in an era of data abundance. While the model’s impressive capabilities demonstrate the potential of generative AI, its misidentification as ChatGPT highlights the challenges of data contamination and ethical considerations in training methodologies.
As the field of AI continues to evolve, addressing these challenges will be crucial to ensuring that models deliver reliable, accurate, and original results. By fostering a culture of transparency and innovation, the AI community can navigate these complexities and unlock new possibilities for technology-driven progress.